Administrative information
Title |
Introduction to Data Privacy |
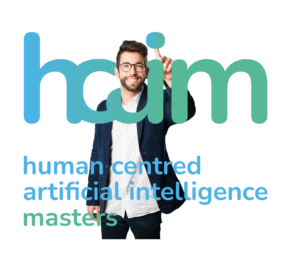 |
Duration |
135 min |
Module |
B |
Lesson Type |
Lecture |
Focus |
Ethical - Trustworthy AI |
Topic |
Data Privacy |
Keywords
Data Privacy, Privacy Risk, Personal data, Sensitive data, Profiling, Tracking, Anonymization, Privacy in Machine Learning, TOR, Pseudonymization, Direct and indirect identifiers,
Learning Goals
- Obtain a general understanding of the notion of privacy.
- Understand the difficulties and pitfalls of data privacy analysis and detection of personal data.
- Understand the trade-off between anonymization and data utility (no free lunch).
- Understand the difference between data security and data privacy.
- Learn the basic principles of anonymous communication and TOR.
- Discern, investigate and discuss key risks which AI and Machine Learning models introduce
Expected Preparation
Learning Events to be Completed Before
Obligatory for Students
- Basic Linear Algebra,
- Basic Machine Learning
Optional for Students
None.
References and background for students:
Lesson Materials
The materials of this learning event are available under CC BY-NC-SA 4.0.
Instructions for Teachers
This lecture provides an overview of data privacy. It focuses on different privacy problems of web tracking, data sharing, and machine learning, as well as some mitigation techniques. The aim is to give the essential (technical) background knowledge needed to identify and protect personal data. The course sheds light on why deriving socially or individually useful information about people is challenging without revealing personal information. These skills are becoming a must of every data/software engineer and data protection officer dealing with personal and sensitive data, and are also required by the European General Data Protection Regulation (GDPR).
Outline
Duration (min) |
Description |
Concepts |
20 |
What is Privacy? |
Privacy as a fundamental right. History or privacy. Importance of privacy. Illustration of data leakage; how much people share about themselve directly or indirectly? Why is privacy a problem? Importance of legislation and technical solutions (PETS). |
15 |
Definition of Personal, Sensitive, Confidential data |
Definition and personal data in GDPR. Direct vs. indirect identifiers. Definition of identifiability. Illustrative examples. Defintion of sensitive data in GDPR, examples. Personal vs sensitive vs confidential data. |
20 |
Illustration of Personal Data Leakage: Tracking |
Purpose of tracking. Web tracking, Browser fingerprinting, WiFi tracking, Ultrasound tracking, Underground tracking through barometer sensor, location inference from battery usage, uniqueness of location data |
20 |
Psychological Profiling |
OCEAN model. Inference of OCEAN personality traits from personal data. Manipulation through personality traits, political ads. Threat of psychological profiling, cognitive security. |
20 |
Anonymization |
Data types, different types need different anonymization techniques. Pseudo-anonymization, de-anonymization, re-identification. Quasi-identifiers, k-anonymity. Generalization, suppression, clusterting as general k-anonymization techniques. Anonymization vs. utility. Impossibility of anonymization without utility loss. Problems of k-anonymization (background knowledge, intersection attack). Anonymization of aggregate data; why aggregation does not prevent re-identification. Query auditing. Auditing SUM queries over reals. Hardness of query auditing. Query perturbation, Differential Privacy. |
20 |
Anonymous communication |
Problem of anonymous communication. Sender, receiver anonymity, unlinkability. Anonymizing proxy. Chaum MIX, MIXnet. TOR, illustration of TOR. Circuit setup in TOR. Exit policies. Some attacks against TOR. |
20 |
Privacy in AI |
Main privacy problems in Machine Learning; membership inference, model extraction, fairness. Source of fairness problems (bias in training data collection/labelling, feature selection, different cultural interpretations of fairness). Protected attributes. Fairness through blindness, redundant encodings (proxy attributes). |
5 |
Conclusions |
Why privacy matters? Why surveillance is a problem? Why everbody has something to hide? Why privacy is hard? What competences does a Data Protection Officer have? Why is there a need for Data Protection Officers? |
More information
Click here for an overview of all lesson plans of the master human centred AI
Please visit the home page of the consortium HCAIM
Acknowledgements
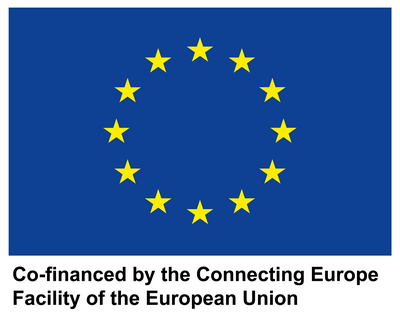 |
The Human-Centered AI Masters programme was co-financed by the Connecting Europe Facility of the European Union Under Grant №CEF-TC-2020-1 Digital Skills 2020-EU-IA-0068.
The materials of this learning event are available under CC BY-NC-SA 4.0
|
The HCAIM consortium consists of three excellence centres, three SMEs and four Universities

|