Administrative information
Title |
Convolutional Neural Networks |
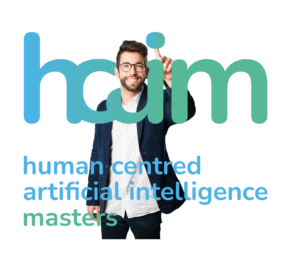 |
Duration |
180 |
Module |
B |
Lesson Type |
Tutorial |
Focus |
Technical - Deep Learning |
Topic |
Deep learning |
Keywords
CNN,Deep learning,Python,
Learning Goals
- Gain experience in training and testing CNNs
- Gain experience in Transfer Learning using CNNs and freezing layers
- Gain experience in a well-known classification problem using CNNs
Expected Preparation
Learning Events to be Completed Before
Obligatory for Students
- Theory and practice on CNN
Optional for Students
References and background for students:
Lesson Materials
The materials of this learning event are available under CC BY-NC-SA 4.0.
Instructions for Teachers
This Practical covers fundamental CNN development, training and testing. Three exercises of increasing difficulty will be administered, each of them covering a different aspect of CNNs. All the proposed solutions will be implemented in Python, using the PyTorch package. The proposed exercises consist in:
- Exercise 1: the simple MNIST dataset will be used to train and test three simple CNNs composed respectively of one, two, and three convolutional layers. Pooling and batch normalisation will be also added to compare the different performances.
- Exercise 2: a deep network (e.g., LeNet-5) pretrained on ImageNet will be loaded. Next, the performances on MNIST and CIFAR10 will be evaluated after a fine-tuning stage. Different experiments will be made, considering different conditions, such as fine-tuning all the layers or only the last ones.
- Exercise 3: the filters of a learned network will be visualized.
- Exercise 4: several datasets (such as CIFAR10 and SVHN) will be tested using other different architectures (such as ResNet and VGG16) and the final performances on the test sets will be evaluated.
Time schedule
Duration (min) |
Description |
Concepts |
Activity |
Material |
40 |
Exercise 1: developing, training and testing simple CNNs on a simple dataset |
|
|
|
40 |
Exercise 2: loading a pre-trained model, evaluation after and before fine-tuning on common datasets |
|
|
|
20 |
Exercise 3: visualizing a subset of learned filters |
|
|
|
80 |
Exercise 3: comparing classification performances on different architectures and more complex data |
|
|
|
|
More information
Click here for an overview of all lesson plans of the master human centred AI
Please visit the home page of the consortium HCAIM
Acknowledgements
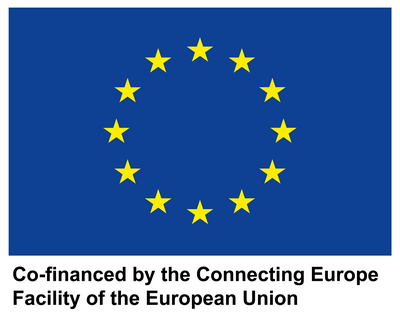 |
The Human-Centered AI Masters programme was co-financed by the Connecting Europe Facility of the European Union Under Grant №CEF-TC-2020-1 Digital Skills 2020-EU-IA-0068.
The materials of this learning event are available under CC BY-NC-SA 4.0
|
The HCAIM consortium consists of three excellence centres, three SMEs and four Universities

|