Administrative information
Title
|
Trust, Normativity and Model Drift |
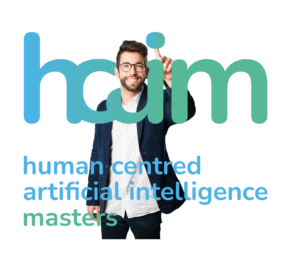 |
Duration |
45-60 |
Module |
C |
Lesson Type |
Lecture |
Focus |
Technical - Future AI |
Topic |
Open Problems and Challenges |
|
Keywords
Trust,Normativity,Model Drift,
Learning Goals
- Understand the need to measure trust in AI
- Understanding the concept of Normativity in context with Future AI
- Explain various kinds of model drift and recommend timely measures for future AI systems to address model drift
Expected Preparation
Learning Events to be Completed Before
Obligatory for Students
- Ethics content from module A
- Ethics content from module B
Optional for Students
- Introduction to machine learning and deep learning concepts given in previous lectures
References and background for students
Recommended for Teachers
None.
Lesson materials
The materials of this learning event are available under CC BY-NC-SA 4.0.
Instructions for Teachers
This lecture should focus on the concept of Trust about systems that employ AI and machine learning to make decisions. It should define trust and the characteristics of trust along with the agents trust. The lecture should provide a practical link to the proposed EU Trust framework for AI. The lecture should also introduce the concept of digital normativity and the problem of model drift, measuring and monitoring model drift in the context of trustworthy AI and machine learning.
The goal of this lecture is to discuss the concept of trust in the context of AI systems. The lecture should answer the question: What does it mean to trust, and how can we build trust in AI systems? The lecture should also discuss the concept of normativity in the context of AI and automated decision making systems, adding weight to the importance of trust. Finally, the lecture will discuss model drift, the types of model drift, metrics to measure model drift, and how to deal with model drift, which should demonstrate that trust should be constantly monitored.
Outline
Duration |
Description |
Concepts |
Activity |
Material |
5 min |
What is trust? |
Philosophy of trust, characterising trust, agents and patients of trust, socio-technical ecosystem, role of trust in knowledge |
Taught session and examples |
Lecture materials |
15 min |
Research Task
Trust in AI
|
Open questions and review of an article |
Lecture materials |
10 min |
Advent of Digital Normativity |
Subjectivation, Desubjectivation, justified agency, explainable and normative agency |
Taught session and examples |
Lecture materials |
15 min |
Model Drift |
What is model drift, types of model/concept drift (prediction, concept, data, upstream), Drift metrics (Population Stability Index, KL divergence, Wasserstein’s Distance), Dealing with model drift (monitoring, data quality, retraining, parameter tuning) |
Taught session and examples |
Lecture materials |
5 min |
Conclusion |
Summary |
Conclusions |
Lecture materials |
More information
Click here for an overview of all lesson plans of the master human centred AI
Please visit the home page of the consortium HCAIM
Acknowledgements
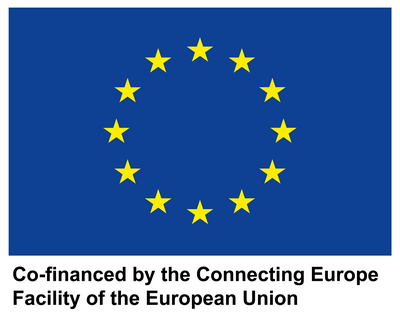 |
The Human-Centered AI Masters programme was co-financed by the Connecting Europe Facility of the European Union Under Grant №CEF-TC-2020-1 Digital Skills 2020-EU-IA-0068.
The materials of this learning event are available under CC BY-NC-SA 4.0
|
The HCAIM consortium consists of three excellence centres, three SMEs and four Universities

|